cloud computing
What Are the Challenges of Edge Computing ?

Introduction
where data processing and storage have become critical for businesses and consumers alike, edge computing has emerged as a powerful solution. Edge computing involves processing data closer to the source or “edge,” of the network, reducing latency and improving overall performance. However, like any technology, edge computing comes with its own set of challenges. In this article, we will explore some of the key obstacles faced in implementing and adopting edge computing solutions
Connectivity and Bandwidth Limitations
One of the primary challenges of edge computing is dealing with limited connectivity and bandwidth in remote or edge locations. These locations might not have access to high-speed internet, making data transmission slower and hindering real-time processing. This constraint requires efficient data compression and optimization techniques to ensure smooth operations at the edge.
Security and Privacy Concerns
Edge devices are often dispersed across various locations, making them vulnerable to security breaches. Protecting these devices from cyber-attacks and unauthorized access becomes a significant challenge. Moreover, edge computing involves processing sensitive data closer to the source, raising concerns about data privacy and compliance with regulations like GDPR.
Edge Device Management
Managing numerous edge devices across different locations can be complex. Configuration, monitoring, and maintenance of these devices require robust management solutions. Ensuring uniformity, updating software, and addressing issues remotely are essential to keep the edge infrastructure running efficiently.
Interoperability
The diversity of edge devices, platforms, and protocols can lead to interoperability challenges. For edge computing to reach its full potential, seamless communication between devices and systems is crucial. The development of standardized interfaces and protocols becomes vital to overcome this challenge.
Scalability
Edge computing deployments need to accommodate rapid growth and scale according to changing requirements. As the number of connected devices and data volume increases, ensuring scalability without compromising performance becomes a significant concern.
Latency
Reducing latency is one of the main reasons for adopting edge computing. However, managing and minimizing latency while processing data closer to the source can be technically demanding. Optimizing data flow and minimizing delays are essential to achieving the desired performance gains.
Edge to Cloud Balance
While edge computing alleviates the burden on the cloud by processing data closer to the source, achieving the right balance between edge and cloud computing can be tricky. Deciding which data should be processed locally and which should be sent to the cloud requires careful planning.
Power and Energy Constraints
Edge devices are often constrained by power sources, especially in remote locations. Energy-efficient computing and low-power devices are necessary to operate effectively in such environments.
Reliability and Redundancy
Edge computing requires high reliability, especially for critical applications. Implementing redundancy and fail-safe mechanisms become essential to ensuring continuous operations even in the face of hardware or network failures.
Edge Analytics and Decision Making
Performing analytics and decision-making at the edge requires efficient algorithms and processing capabilities. Ensuring that edge devices can analyze data effectively and make intelligent decisions without relying heavily on the cloud is a challenge.
Cost Considerations
Implementing edge computing solutions can involve substantial costs, particularly when deploying devices across multiple locations. Businesses must carefully weigh the benefits against the expenses to make informed decisions.
Regulatory Compliance
As edge devices often handle sensitive data, adhering to various industry regulations and data protection laws becomes crucial. Navigating through these regulatory requirements can be a challenge for organizations adopting edge computing
Regulatory Compliance
As edge devices often handle sensitive data, adhering to various industry regulations and data protection laws becomes crucial. Navigating through these regulatory requirements can be a challenge for organizations adopting edge computing.
Skill Gaps and Training
Edge computing requires specialized skills to manage and maintain the infrastructure effectively. Organizations need to invest in training their staff or hiring experts in edge computing technologies.
Integration with Existing Systems
Integrating edge computing with existing IT systems can be complex. Ensuring seamless compatibility and avoiding disruptions to the current workflow is essential during the implementation phase.
Data Synchronization and Consistency
Keeping data consistent across edge devices and the central cloud system can be challenging, especially in scenarios where devices frequently go offline or experience network issues.
Conclusion
Edge computing offers exciting opportunities for businesses to improve data processing, reduce latency, and enhance overall performance. However, successful adoption requires overcoming several challenges, including connectivity limitations, security concerns, scalability, and regulatory compliance. By addressing these obstacles and strategically implementing edge computing solutions, organizations can unlock the full potential of this transformative technology.
FAQs About Edge Computing
- What is the main advantage of edge computing?
Edge computing significantly reduces data processing and transmission latency by bringing computation closer to the source of data, resulting in faster response times and improved performance.
- How does edge computing enhance data security?
Edge computing reduces the attack surface by processing data locally, making it harder for hackers to target centralized cloud systems. It also allows for real-time data analysis, enabling quick identification of potential security breaches.
- Can edge computing work without an internet connection?
Yes, edge computing can function without an internet connection. It processes data locally, making it suitable for edge locations with limited or no connectivity.
- What industries can benefit the most from edge computing?
Industries like healthcare, manufacturing, transportation, and IoT-based applications can benefit significantly from edge computing due to their reliance on real-time data processing and low-latency requirements.
- Is edge computing a replacement for cloud computing?
No, edge computing complements cloud computing by offloading certain tasks and reducing data transmission to the cloud. Both technologies work together to create a comprehensive and efficient computing ecosystem.
cloud computing
Edge Computing: Revolutionizing Data Processing and Analysis
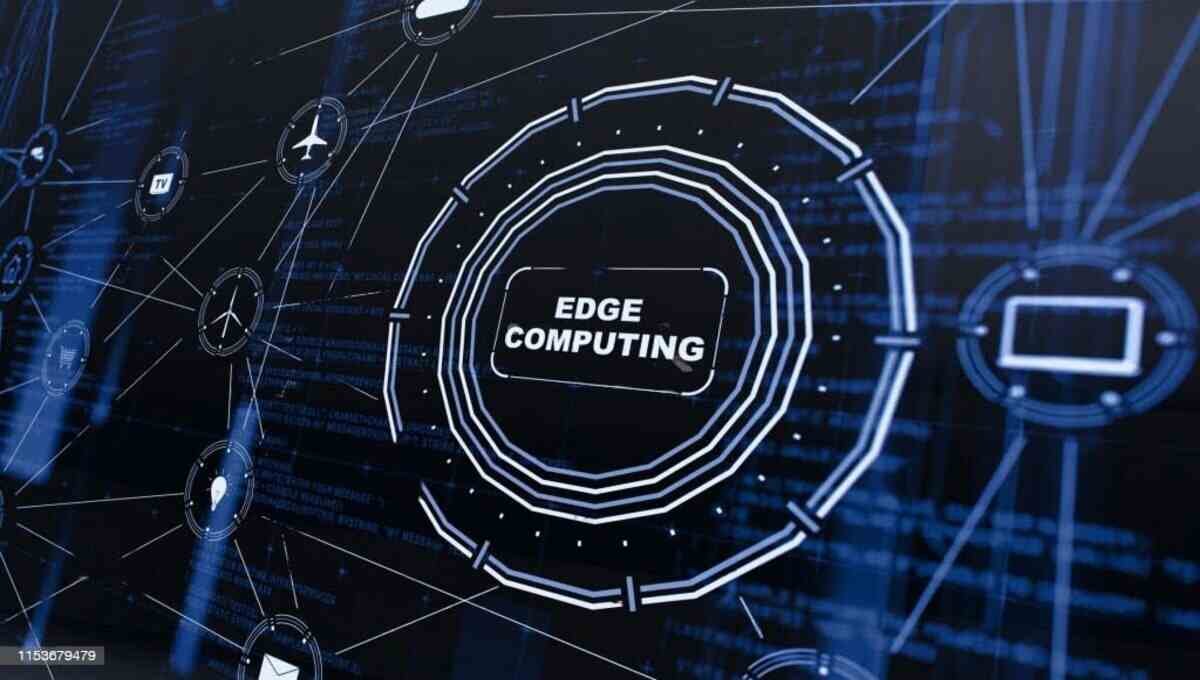
Edge Computing
Businesses and individuals are continuously looking for efficient ways to manage and analyze the enormous amount of data that is generated in the modern digital age. Edge computing has emerged as a promising solution to address this need. In this article, we will explore the concept of edge computing, its benefits, and its potential applications across various industries.
Understanding the Basics
What is Edge Computing?
Edge computing refers to the decentralized computing infrastructure that allows data processing and analysis to occur near the Edge of the network, where the data is generated or consumed. Unlike traditional cloud computing, where data travels back and forth between devices and remote data centers, edge computing brings computation closer to the data source.
How does edge computing work?
Edge computing leverages a network of edge devices, including routers, gateways, servers, and IoT devices, to process and analyze data locally. These edge devices act as mini-data centers, capable of executing tasks and running applications without relying heavily on cloud infrastructure. By reducing the distance data needs to travel, edge computing minimizes latency and optimizes bandwidth usage.
Critical Components of Edge Computing
An edge computing ecosystem’s key components include edge devices, servers, gateways, and analytics platforms. Edge devices, like sensors or smart devices, capture and generate data. Edge servers and gateways enable data processing, storage, and communication. Edge analytics platforms provide the necessary tools and software to analyze and extract insights from the data collected at the Edge.
Advantages of Edge Computing
- Improved performance and latency: Edge computing reduces the distance that data has to travel, which can significantly improve performance and latency for real-time applications.
- Reduced bandwidth usage: Edge computing can help to reduce bandwidth usage by processing data closer to the source, which can be especially beneficial for applications that generate large amounts of data.
- Improved reliability and security: Edge computing can help to improve reliability and security by distributing data and processing across multiple devices. This makes it less likely that a single failure will disrupt the entire system, and it also makes it more difficult for attackers to steal data.
- Reduced costs: Edge computing can help to reduce costs by reducing bandwidth usage and by eliminating the need to send all data to a central data center.
- Increased scalability and flexibility: Edge computing makes it easier to scale and adapt IT infrastructure to meet changing needs. This is because edge devices can be added or removed as needed, and they can be configured to perform a variety of different tasks.
Disadvantages of Edge Computing
- Increased complexity: Edge computing can add complexity to IT infrastructure, as it requires the management of a distributed network of devices.
- Security challenges: Edge computing can introduce new security challenges, as edge devices are often more vulnerable to attack than central data centers.
- Cost of hardware and software: The cost of edge hardware and software can be significant, especially for large deployments.
- Lack of skilled workers: There is a shortage of skilled workers who have the expertise to design, implement, and manage edge computing systems.
Overall, edge computing offers a number of advantages, including improved performance, latency, reliability, security, and cost savings. However, it is important to weigh the advantages against the disadvantages before deciding whether to implement edge computing.
Use Cases of Edge Computing
Internet of Things (IoT)
Edge computing plays a pivotal role in the success of the Internet of Things (IoT). IoT devices can operate in real-time by processing data at the edge and making rapid decisions based on localized analytics. This enables efficient monitoring, control, and automation of various systems, including smart homes, industrial sensors, and environmental monitoring.
Autonomous Vehicles
Edge computing is a fundamental component of autonomous vehicles. The enormous amount of data generated by self-driving cars in sensors, cameras, and radar systems requires real-time processing and decision-making capabilities. Edge computing enables autonomous vehicles to make split-second decisions without relying solely on cloud connectivity, ensuring safe and efficient operation.
Smart Cities
Edge computing empowers the development of smart cities by enabling distributed intelligence and efficient urban infrastructure management. From traffic management and public safety to waste management and energy optimization, edge computing allows real-time data analysis and decision-making, enhancing the overall quality of urban living.
Healthcare
Edge computing has transformative potential in healthcare applications. By bringing data processing and analysis closer to medical devices and sensors, critical patient information can be analyzed in real-time, allowing for faster diagnosis, remote patient monitoring, and improved healthcare outcomes. Edge computing also addresses data privacy and security concerns in the healthcare sector.
Challenges and Considerations
Scalability
Scaling edge computing systems can be challenging due to the distributed nature of the infrastructure. Coordinating and managing many edge devices, ensuring seamless communication, and dynamically allocating resources require careful planning and efficient orchestration.
Network Connectivity
Edge computing relies on reliable network connectivity between edge devices and the central cloud infrastructure. Ensuring seamless operation and synchronization can be complex in areas with poor network coverage or intermittent connectivity.
Data Management
Managing data at the Edge presents unique challenges. Ensuring data integrity, consistency, and synchronization across multiple edge devices requires robust data management strategies. Additionally, dealing with the large volumes of data generated at the Edge requires efficient storage and processing capabilities.
Security Risks
Edge computing introduces new security risks, such as device tampering, unauthorized access, and data breaches. Implementing robust security measures, including encryption, authentication, and access controls, is crucial to mitigate these risks and safeguard critical data.
Future Trends and Innovations
The future of edge computing is poised for significant advancement. Edge AI, where artificial intelligence algorithms are deployed at the edge, will enable more intelligent and autonomous edge devices. The integration of 5G networks will enhance the capabilities of edge computing by providing high-speed, low-latency connectivity. Additionally, advancements in edge analytics and machine learning techniques will enable more sophisticated data processing and decision-making at the edge.
Conclusion
Edge computing has emerged as a powerful paradigm that brings computing capabilities closer to the source of data generation. With reduced latency, enhanced security, optimized bandwidth usage, and improved reliability, edge computing offers numerous benefits across various industries. From IoT and autonomous vehicles to smart cities and healthcare, edge computing is revolutionizing how we process, analyze, and utilize data. As technology continues to advance, edge computing is set to play a vital role in shaping the future of the digital landscape.
Frequently Asked Questions (FAQs)
- How is data processed in edge computing?
Edge computing is a distributed information technology (IT) architecture in which client data is processed as near to the original source as is practical at the network’s edge.
- What is edge computing data analytics?
Instead of sharing time-sensitive, secret, or proprietary information over a weak network, edge analytics offers smoother, safer usage of data. Furthermore, the price of cloud computing, transfer bandwidth, and data storage can quickly run into thousands of dollars each day.
- What are the major two types of edge data?
There are two major types of edge data centers, namely metro edge facilities, which are located in suburban markets, and mobile edge facilities, which are deployed in C-RAN (Cloud-Radio Access Network) hubs and at the base of cell towers.
- What are the benefits of data processing at the edge?
Data is processed and stored locally via edge computing. As a result, there is less need for data to travel to and from the cloud. Additionally, decreasing data transit reduces the risk of data compromise since Edge computing offers fewer possibilities to attack sensitive data while it is being transmitted.
- What are some of the future trends in edge computing?
The integration of AI at the edge.
Deploying 5G networks for enhanced connectivity.
Advancing edge analytics and machine learning techniques.
cloud computing
What is GPU computing? All you need to know
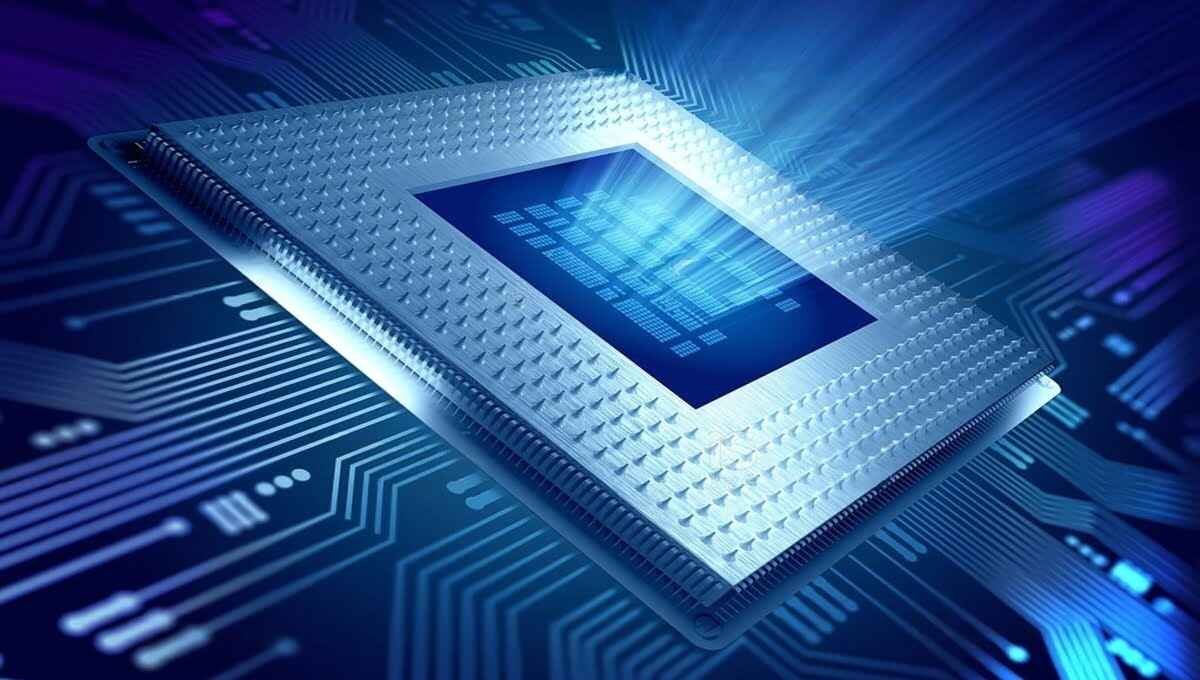
GPU computing, or general-purpose computing on graphics processing units, is the use of a GPU to perform tasks that were traditionally handled by the CPU. GPUs are highly parallel processors that can perform millions of calculations simultaneously, making them ideal for tasks that can be broken down into small, independent tasks.
How does GPU cloud computing work?
GPU cloud computing is a service that allows users to access and use GPUs on demand from a cloud provider. This means that users do not need to purchase and maintain their own GPUs, which can be expensive and time-consuming. Instead, they can simply rent GPUs as needed from the cloud provider.
To use GPU cloud computing, users first need to create an account with a cloud provider that offers GPU services. Once they have an account, they can then select the type and number of GPUs they need. The cloud provider will then create a virtual machine with the specified GPUs and make it available to the user.
The user can then use the virtual machine to run their applications on the GPUs. The GPUs will provide a significant boost in performance for applications that are designed to take advantage of parallel computing.
ome of the applications of GPU computing include:
- Machine learning: GPUs are used to train and deploy machine learning models, which are used for tasks such as image recognition, natural language processing, and fraud detection.
- Data science: GPUs are used to analyze large datasets, which is essential for tasks such as data mining and predictive analytics.
- Scientific computing: GPUs are used to solve complex scientific problems, such as climate modeling and protein folding.
- Graphics: GPUs are used to render graphics in real time, which is essential for gaming and video editing.
- Cryptocurrency mining: GPUs are used to mine cryptocurrency, which is a process of verifying and adding new transactions to a blockchain.
GPU computing is a rapidly growing field, and it is becoming increasingly common for businesses and individuals to use GPUs to improve the performance of their applications.
What programming language is used for GPUs?
There are several programming languages that can be used for GPU programming, but the most popular ones are:
- CUDA: CUDA is a proprietary programming language developed by NVIDIA. It is designed specifically for GPU programming and provides a high level of performance.Opens in a new windowblogs.nvidia.com CUDA programming language logo
- OpenCL: OpenCL is an open standard for parallel programming of heterogeneous systems. It can be used to program GPUs, CPUs, and other accelerators.Opens in a new windowen.wikipedia.org OpenCL programming language logo
- HIP: HIP is a C++ runtime API and kernel language developed by AMD. It is similar to CUDA, but it can be used to program both AMD and NVIDIA GPUs.Opens in a new windowwww.pxfuel.comHIP programming language logo
- SYCL: SYCL is a C++ abstraction layer for OpenCL developed by the Khronos Group. It makes it easier to write code that can be run on both CPUs and GPUs.Opens in a new windowen.wikipedia.org SYCL programming language logo
- Python: Python is a general-purpose programming language that can also be used for GPU programming. There are several libraries available that make it easy to write GPU-accelerated Python code.Opens in a new windowen.wikipedia.org Python programming language logo
The best programming language for GPU programming depends on the specific application and the needs of the developer. For example, if you are developing an application that will only run on NVIDIA GPUs, then CUDA is a good choice. If you need to run your code on a variety of hardware platforms, then OpenCL may be a better choice.
If you are new to GPU programming, I recommend starting with Python. There are many resources available to help you learn how to write GPU-accelerated Python code. Once you have a good understanding of the basics, you can then explore other programming languages such as CUDA and OpenCL.
Here are some of the benefits of GPU cloud computing:
- Cost-effectiveness: GPU cloud computing is a cost-effective way to access high-performance GPUs. Users only pay for the GPUs they use, which can save them a significant amount of money compared to purchasing and maintaining their own GPUs.
- Scalability: GPU cloud computing is scalable, so users can easily add or remove GPUs as needed. This makes it ideal for applications that have fluctuating or unpredictable workloads.
- Flexibility: GPU cloud computing is flexible, so users can access GPUs from anywhere with an internet connection. This makes it ideal for businesses and individuals who need to use GPUs on a temporary basis.
Here are some of the drawbacks of GPU cloud computing:
- Latency: There can be some latency when using GPU cloud computing, as the data needs to travel over the internet to reach the GPUs. This can be a problem for applications that require real-time processing.
- Security: Security is a concern with any cloud computing service, including GPU cloud computing. Users need to make sure that they are using a reputable cloud provider that has strong security measures in place.
Is GPU important for coding?
A GPU (Graphics Processing Unit) is not typically necessary for coding. Coding does not typically require a lot of graphical processing power, and most tasks can be performed effectively with an integrated graphics processor or a relatively low-end dedicated graphics card.
Here are some of the factors to consider when deciding whether or not you need a GPU for coding:
- The type of programming you do: If you are doing mostly general-purpose programming, then you do not need a GPU. However, if you are doing any of the tasks listed above, then a GPU can be helpful.
- Your budget: GPUs can be expensive, so you need to decide if the cost is worth it for the tasks you will be doing.
- Your computer’s specifications: If your computer has a built-in GPU, then you may not need to purchase a dedicated GPU. However, if your computer does not have a GPU, then you will need to purchase one.
Ultimately, the decision of whether or not to get a GPU for coding is up to you. Consider the factors above and decide what is best for your needs.
conclusion
GPU computing is a rapidly growing field that is being used in a wide variety of applications. GPUs are highly parallel processors that can perform millions of calculations simultaneously, making them ideal for tasks that can be broken down into small, independent tasks.
FAQs
How is GPU calculated?
You must rewrite this function in Metal Shading Language (MSL) to do the calculation on the GPU. MSL is a C++ subset created specifically for GPU programming. Since historically they were first used to calculate colors in 3D graphics, GPU-based code is referred to as a shadier in the Metal programming language.
How much GPU is enough for programming?
Even though programming does not require a dedicated graphics card, running simulations, animations, and visual design software can benefit from one. For programming requirements, the Intel Iris Xe Graphics or NVIDIA GeForce RTX 3050/3050 Ti are excellent choices [3].
Who invented GPU?
Nvidia, however, is recognized as the GPU’s inventor and is credited with popularizing the word. The 120 MHz NV10 used DirectX 7.0 and has 17 million transistors packed into a 139 mm2 die. It was manufactured using TSMC’s 220 nm technology.
cloud computing
Why GPU is Good for Machine Learning

Introduction
In the rapidly evolving field of machine learning, the role of Graphics Processing Units (GPUs) has become increasingly vital. GPUs, which were initially designed for rendering graphics in video games, have proven to be a game-changer for training and optimizing machine learning models. In this article, we’ll explore the reasons why GPUs are so effective in the realm of machine learning.
The Power of Parallelism
Harnessing Parallel Processing
One of the primary reasons GPUs excel in machine learning is their ability to perform parallel processing. Unlike Central Processing Units (CPUs), which excel at sequential tasks, GPUs can simultaneously execute multiple computations. This is crucial for machine learning tasks that require performing complex mathematical operations on massive datasets.
Faster Training
By utilizing parallel processing, GPUs significantly expedite the training of machine learning models. Tasks that would take days or even weeks to complete on CPUs can be done in a matter of hours with GPUs. This accelerated training process allows researchers and practitioners to iterate and experiment with their models more efficiently.
Optimized for Matrix Operations
Matrix Multiplications
Matrix operations are at the heart of many machine learning algorithms. GPUs are well-suited for these operations due to their architecture, which is designed to handle these computations efficiently. This makes GPUs particularly effective for tasks like convolutional neural networks (CNNs) used in image recognition, where matrix multiplications are prevalent.
Deep Learning Advantage
Deep learning models, characterized by their complex neural architectures, heavily rely on matrix operations during both forward and backward propagation. GPUs’ prowess in matrix calculations directly translates to faster and more efficient training of deep learning models.
Memory Bandwidth and Speed
High Memory Bandwidth
GPUs are equipped with high memory bandwidth, allowing them to read and write data from and to memory at a rapid pace. This is crucial for machine learning workloads that involve frequent data transfers between the processor and memory.
Data-Intensive Tasks
Machine learning often involves processing vast amounts of data. GPUs’ high memory bandwidth enables them to handle these data-intensive tasks without causing bottlenecks, resulting in smoother and faster execution.
GPU Libraries and Frameworks
CUDA and cuDNN
NVIDIA’s CUDA (Compute Unified Device Architecture) platform and cuDNN (CUDA Deep Neural Network) library provide developers with tools to optimize and accelerate machine learning algorithms on GPUs. These libraries offer specialized functions that leverage the GPU’s capabilities for faster computations.
TensorFlow and PyTorch
Popular machine learning frameworks like TensorFlow and PyTorch have GPU support, allowing practitioners to seamlessly integrate GPUs into their workflow. This compatibility empowers researchers to experiment with complex models and large datasets more efficiently.
Energy Efficiency
Performance per Watt
GPUs not only deliver exceptional performance but also do so in an energy-efficient manner. This is especially important in today’s environmentally conscious landscape, where minimizing energy consumption is a top priority.
Reduced Carbon Footprint
Using GPUs for machine learning can contribute to reducing the carbon footprint associated with data centers and large-scale computations. Their energy efficiency allows for more work to be done with less power, ultimately benefiting the environment.
CONCLUSION
In conclusion, the role of GPUs in advancing machine learning cannot be overstated. Their parallel processing capabilities, optimized matrix operations, high memory bandwidth, and energy efficiency make them an indispensable tool for researchers and practitioners alike. As machine learning continues to evolve, GPUs will undoubtedly remain a driving force behind its progress.
FAQs (Frequently Asked Questions)
Yes, many modern GPUs, especially those from NVIDIA and AMD, are suitable for machine learning tasks. However, high-end GPUs with greater computational power are often preferred for more demanding tasks.
Yes, many modern GPUs, especially those from NVIDIA and AMD, are suitable for machine learning tasks. However, high-end GPUs with greater computational power are often preferred for more demanding tasks.
No, GPUs can accelerate a wide range of machine learning tasks, including but not limited to deep learning. Tasks involving large datasets and complex computations can benefit from GPU acceleration.
No, besides GPUs, other hardware like Field-Programmable Gate Arrays (FPGAs) and Application-Specific Integrated Circuits (ASICs) is also utilized in certain machine learning applications.
While GPUs offer significant advantages, they can be expensive to acquire and may require additional cooling solutions to prevent overheating in prolonged computations.
- 5G1 year ago
How 5G Technology Will Revolutionize Our Lives and Work
- Tech4 months ago
3d Printer Technology (Application) (History) And (Types)
- 5G1 week ago
What is the difference between 5G and 5G Plus?
- Computer1 year ago
“Bleeping Computer: Your Ultimate Guide to Cybersecurity”
- Tech1 year ago
Explain How Technology Has Affected People’s Activity Levels
- 5G1 year ago
Difference between 5G nsa and 5G sa
- cloud computing4 months ago
Edge Computing: Revolutionizing Data Processing and Analysis
- Computer1 year ago
What is a Server on the Internet and How It Works